
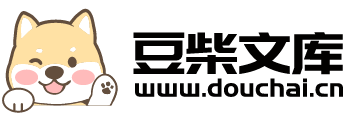
基于AE和Transformer的运动想象脑电信号分类研究.docx
骑着****猪猪
在线预览结束,喜欢就下载吧,查找使用更方便
相关资料
基于AE和Transformer的运动想象脑电信号分类研究.docx
基于AE和Transformer的运动想象脑电信号分类研究AbstractTheclassificationofmotorimageryEEGsignalshasbecomeanimportantresearchtopicinthefieldofbrain-computerinterfaces(BCIs)duetoitspotentialapplicationsinprostheticsandrehabilitation.Inrecentyears,deeplearningmodelssuchasthea
基于LDA和KNN的下肢运动想象脑电信号分类研究.docx
基于LDA和KNN的下肢运动想象脑电信号分类研究摘要:下肢运动想象脑电信号是一种非常重要的生物信号,其能够反映出人体下肢运动的相关信息,因此在很多领域中具有广泛的应用。本文以下肢运动想象脑电信号分类为主题,结合LDA和KNN算法进行研究,分别从特征提取与分类两个方面进行介绍。首先,通过多种特征提取方法进行比较,选择最具代表性的特征提取方式作为后续分类的依据;其次,基于所选定的特征提取方式,探究不同参数对于KNN分类效果的影响,并确定最佳参数组合;最后根据实验结果进行总结和分析,为研究者提供一定参考价值。关
基于深度学习的运动想象脑电信号分类算法研究.docx
基于深度学习的运动想象脑电信号分类算法研究论文题目:基于深度学习的运动想象脑电信号分类算法研究摘要:运动想象是人类运动神经系统的一种特殊能力,在脑电信号分类领域有着广泛的应用。本研究基于深度学习的方法,对运动想象脑电信号进行分类,以提高分类准确度和实时性。首先,采集运动想象脑电信号并进行预处理,包括滤波、分段和特征提取。然后,使用卷积神经网络(CNN)进行特征学习和分类。通过对比实验,验证所提方法在运动想象脑电信号分类任务上的有效性。实验结果表明,基于深度学习的运动想象脑电信号分类算法能够在高分类准确度和
基于CSP和RR的多类运动想象脑电信号的识别分类研究.docx
基于CSP和RR的多类运动想象脑电信号的识别分类研究基于CSP和RR的多类运动想象脑电信号的识别分类研究摘要:脑电信号的识别分类在脑机接口技术中起着重要的作用,尤其是对于多类运动想象的脑电信号分类的研究具有重要的意义。本文主要基于CSP(CommonSpatialPatterns)和RR(RiemannianGeometryRepresentation)的方法,研究了多类运动想象脑电信号的识别分类。通过实验数据的采集和信号处理,本文对CSP和RR方法的原理、步骤及其在多类运动想象脑电信号分类中的应用进行了
脑-机接口中运动想象脑电信号的分类研究.docx
脑-机接口中运动想象脑电信号的分类研究脑-机接口(Brain-ComputerInterface,BCI)是一种将人类大脑信号转换为计算机控制命令的技术。运动想象(MotorImagery,MI)指的是在没有实际运动的情况下想象强烈的动作。MI是BCI应用领域中应用最为广泛的一种信号。运动想象脑电信号的分类是BCI应用的重要研究内容之一,本文旨在对该方向的研究进行概述和分析。一、脑-机接口技术的发展传统的遥控器、鼠标、键盘等控制设备,需要肌肉的参与来控制。而肌肉运动的控制指令是由大脑通过神经系统传递给肌肉