
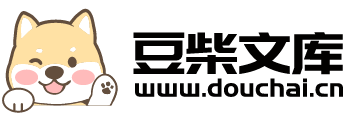
基于卷积神经网络和迁移学习的结构损伤识别.docx
骑着****猪猪
在线预览结束,喜欢就下载吧,查找使用更方便
相关资料
基于卷积神经网络和迁移学习的结构损伤识别.docx
基于卷积神经网络和迁移学习的结构损伤识别AbstractWiththedevelopmentofintelligentdetectiontechnology,theapplicationofstructuraldamagerecognitionhasbeenfurtherexpanded.However,traditionalstructuraldamagerecognitionmethodshavesomeshortcomings,suchasthedifficultyinfeatureextracti
基于卷积神经网络的结构损伤识别.docx
基于卷积神经网络的结构损伤识别概述结构损伤识别是一个重要的领域,它可以应用于工业领域、航空领域、建筑领域等。基于卷积神经网络的结构损伤识别是目前研究方向之一。卷积神经网络是一种深度学习模型,由于其良好的特征提取能力和自适应学习能力,已经在图像识别、语音识别等领域取得了很大的成功。本文将从卷积神经网络的基本概念开始,介绍卷积神经网络在结构损伤识别中的应用,并对卷积神经网络在结构损伤识别中的优缺点进行了分析。卷积神经网络的基本概念卷积神经网络是一种常用于模式识别、语音识别和图像识别等领域的计算模型。它模拟了人
基于卷积神经网络和迁移学习的钢桥病害识别.pptx
汇报人:/目录0102卷积神经网络的基本原理迁移学习的概念及应用钢桥病害识别的意义03图像预处理技术卷积神经网络模型构建训练与优化识别精度评估04迁移学习的基本流程预训练模型的选择与微调自适应调整网络参数提高识别准确率的策略05实验数据集介绍实验过程与结果展示结果分析对比对未来研究的建议06钢桥病害的实地调查与数据采集基于卷积神经网络和迁移学习的病害识别系统设计系统实现与测试效果对实际工程的意义与价值07研究成果总结未来研究方向展望汇报人:
基于一维卷积神经网络的结构损伤识别.docx
基于一维卷积神经网络的结构损伤识别摘要:结构损伤识别是结构健康监测领域中的研究热点之一。随着传感器技术和数据处理技术的不断发展,基于传感器数据的结构损伤识别方法不断被提出,其中基于卷积神经网络的方法受到了广泛关注。本文提出了一种基于一维卷积神经网络的结构损伤识别方法。使用了公共场景分解算法对传感器数据进行降维处理,并将处理后的数据作为网络输入。实验结果表明,该方法能够准确识别出不同类型的结构损伤。关键词:结构损伤识别,一维卷积神经网络,公共场景分解算法1.研究背景与意义随着城市化程度的不断提高,建筑物的数
基于迁移学习和卷积神经网络的二维形状识别.docx
基于迁移学习和卷积神经网络的二维形状识别基于迁移学习和卷积神经网络的二维形状识别摘要:近年来,深度学习在计算机视觉领域取得了显著的进展。迁移学习作为一种广泛应用的深度学习技术,已被证明对于小样本二维形状识别任务具有很大的帮助。本论文通过引入迁移学习的方法,结合卷积神经网络,实现了对二维形状的识别。实验结果表明,迁移学习可以有效提高模型的识别准确率,为二维形状识别任务提供了一个可行的解决方案。关键词:迁移学习;卷积神经网络;二维形状识别1.引言在计算机视觉领域,形状识别一直是一个重要的研究方向。随着深度学习