
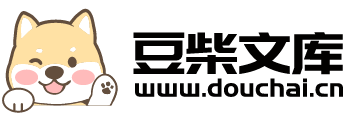
DSP指令集仿真器的优化方案研究的中期报告.docx
骑着****猪猪
在线预览结束,喜欢就下载吧,查找使用更方便
相关资料
DSP指令集仿真器的优化方案研究的中期报告.docx
DSP指令集仿真器的优化方案研究的中期报告AbstractThisreportpresentstheresearchprogressofoptimizingDSPinstructionsetsimulator.Thereportfirstintroducesthebackgroundandsignificanceoftheresearch,andthenreviewstherelatedwork.Followingthat,thereportdescribestheproblemsandchallenge
DSP指令集仿真器的优化方案研究的开题报告.docx
DSP指令集仿真器的优化方案研究的开题报告一、选题背景DSP(DigitalSignalProcessor,数字信号处理器)是一类专用于数字信号处理的微处理器,广泛应用于音频、视频、图像、通信、雷达等领域。DSP指令集仿真器可以将DSP指令集在通用处理器上实现仿真,从而在硬件设计和软件开发过程中提供实时仿真的功能。仿真器的效率和性能对于设计和开发过程的效率和质量具有重要影响。二、研究目的本研究的主要目的是针对DSP指令集仿真器的性能进行优化,以提高仿真效率和精度。具体任务包括:(1)研究当前常用的DSP指
DSP指令集仿真器的优化方案研究的任务书.docx
DSP指令集仿真器的优化方案研究的任务书任务书一、背景DSP(DigitalSignalProcessing)芯片是一种专门用于数字信号处理的芯片,主要应用于通信、音视频处理等领域。在DSP芯片的开发过程中,需要对DSP芯片的指令集进行仿真,以确保其符合要求,同时提高开发效率。DSP指令集仿真器是一种用于模拟DSP芯片执行指令的软件,能够帮助开发人员进行指令级别的仿真。然而,在实际使用过程中,由于指令集较大,且执行过程中需要考虑多种因素,因此仿真器效率低,易导致仿真时间过长,影响开发进程。因此,本研究旨在
ARM指令集仿真器的设计与实现的中期报告.docx
ARM指令集仿真器的设计与实现的中期报告这篇中期报告将会从以下四个方面介绍ARM指令集仿真器的设计与实现:1.实现目标2.已完成的工作3.面临的挑战4.后续计划##1.实现目标我们的目标是设计并实现一个能够运行大部分ARM指令的仿真器,该仿真器应该能够:-读取ARM汇编代码文件并解析指令-模拟ARM指令的逻辑执行-处理内存和寄存器的读写操作-支持单步调试和断点功能-提供基本的性能统计信息##2.已完成的工作目前我们已经完成了以下工作:-实现了ARM指令集的解析器,包括处理数据处理指令(DataProces
指令集仿真器自动生成技术及其优化.docx
指令集仿真器自动生成技术及其优化指令集仿真器自动生成技术及其优化摘要:随着计算机技术的快速发展,指令集架构的设计和优化变得日益重要。指令集仿真器作为一种关键的工具,用于模拟和测试计算机指令集的行为和性能。传统的指令集仿真器的开发需要大量的人工参与和耗费大量的时间和资源,且容易受制于人为因素,导致仿真器的质量和性能不能得到有效保证。为了解决这个问题,自动化生成指令集仿真器的技术应运而生。本文将介绍指令集仿真器自动生成技术的研究进展和优化方法,并讨论其在指令集架构设计和性能优化中的应用。一、引言指令集架构是计