
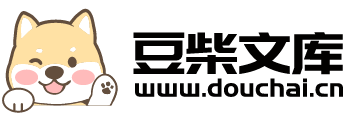
基于高斯混合模型的改进的减法聚类算法.docx
快乐****蜜蜂
在线预览结束,喜欢就下载吧,查找使用更方便
相关资料
基于高斯混合模型的改进的减法聚类算法.docx
基于高斯混合模型的改进的减法聚类算法IntroductionGaussianMixtureModel(GMM)isastatisticalmodelusedinunsupervisedlearningforrepresentingthedistributionofdatainamulti-dimensionalspace.ThemodelassumesthateachdatapointisgeneratedfromamixtureofGaussiandistributionswithunknownpara
基于高斯混合模型EM聚类算法的研究及应用.docx
基于高斯混合模型EM聚类算法的研究及应用基于高斯混合模型EM聚类算法的研究及应用摘要:随着数据规模的不断增大,聚类算法在数据挖掘和机器学习领域中日益受到重视。高斯混合模型(GaussianMixtureModel,GMM)是一种常用的聚类算法,具有较好的建模能力和灵活性。本文综述了基于高斯混合模型的EM聚类算法的研究进展,并探讨了其在实际应用中的潜在价值。1.引言聚类算法是一种将相似样本归类为同一类别的方法,已广泛应用于数据挖掘、模式识别和图像处理等领域。高斯混合模型是一种常用的聚类算法,它假设每个类别是
基于K-均值的混合高斯模型聚类算法研究.docx
基于K-均值的混合高斯模型聚类算法研究基于K-均值的混合高斯模型聚类算法研究摘要:聚类算法是数据挖掘领域中的一项重要任务,对于大规模数据的处理和分析具有很大的意义。其中,基于K-均值算法的混合高斯模型聚类算法是一种常用且有效的聚类方法。本文主要研究了基于K-均值的混合高斯模型聚类算法的原理、优缺点及应用,并通过案例分析来验证其有效性。关键词:聚类算法;K-均值算法;混合高斯模型;优缺点;应用一、引言随着数据挖掘技术的不断发展,聚类算法作为数据挖掘的一项基础性任务,受到了广泛关注。聚类算法的目标是将数据集划
基于改进混合高斯模型的前目标提取算法.docx
基于改进混合高斯模型的前目标提取算法基于改进混合高斯模型的前目标提取算法摘要:前目标提取是计算机视觉和图像处理领域的重要研究内容之一,它在目标跟踪、行人检测等应用中发挥着重要的作用。然而,传统的前目标提取算法往往受到光照变化、遮挡等因素影响。为了解决这些问题,本文提出了一种基于改进混合高斯模型的前目标提取算法。首先,通过采集一系列不同场景下的图像,建立起混合高斯模型来描述背景信息。然后,根据当前帧图像与背景模型之间的差异,采用自适应阈值来分割前景目标。最后,通过形态学处理和图像增强技术,进一步提取目标边界
高斯混合模型聚类.docx
高斯混合模型详解聚类的方法有很多种,k-means要数最简单的一种聚类方法了,其大致思想就是把数据分为多个堆,每个堆就是一类。每个堆都有一个聚类中心(学习的结果就是获得这k个聚类中心),这个中心就是这个类中所有数据的均值,而这个堆中所有的点到该类的聚类中心都小于到其他类的聚类中心(分类的过程就是将未知数据对这k个聚类中心进行比较的过程,离谁近就是谁)。其实k-means算的上最直观、最方便理解的一种聚类方式了,原则就是把最像的数据分在一起,而“像”这个定义由我们来完成,比如说欧式距离的最小,等等。想对k-