
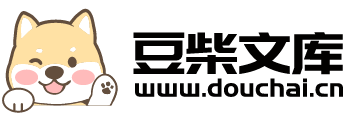
基于Context模型和矢量—标量量化器的ECG信号压缩.docx
快乐****蜜蜂
在线预览结束,喜欢就下载吧,查找使用更方便
相关资料
基于Context模型和矢量—标量量化器的ECG信号压缩.docx
基于Context模型和矢量—标量量化器的ECG信号压缩Abstract:ECG(Electrocardiogram)signalcompressionreferstotheprocessofreducingthesizeoftheECGsignalwhileretainingtheimportantinformation.Inthispaper,weproposeanovelmethodtocompressECGsignalsbasedontheContextmodelandVector-ScalarQ
基于嵌入式量化器和自适应Context建模的ECG信号压缩.docx
基于嵌入式量化器和自适应Context建模的ECG信号压缩1.引言随着心脏病和其他心血管疾病的普及,对心电图(ECG)信号分析的需求越来越高。但是,ECG数据的大小和数量也随着该趋势而增加,这导致了存储和传输的问题。因此,ECG信号压缩作为一种必要的技术得到广泛关注。本文提出了一种基于嵌入式量化器和自适应Context建模的ECG信号压缩方法。2.相关工作ECG信号压缩算法主要分为两类:无损压缩和有损压缩。无损压缩算法可以压缩ECG信号,而不会对信号的完整性产生任何影响。经典的无损压缩算法包括无损压缩(L
基于Context模型的ECG信号二维压缩.docx
基于Context模型的ECG信号二维压缩摘要:心电图(ECG)信号在临床中具有重要的价值,但信号的数据量大,处理难度高,这对信号处理技术提出了新的挑战。在本文中,我们将探讨一种基于Context模型的ECG信号二维压缩方法。该方法将ECG信号处理成二维图像,然后采用Context模型进行压缩。首先,我们将ECG信号处理成二维图像,这里我们采用了连续小波变换(CWT)的方法。通过CWT,我们可以将ECG信号分解成多个尺度和频率,然后将其转化为二维图像。其次,我们提出了一种基于Context模型的压缩算法。
基于嵌入式量化器和自适应Context建模的ECG信号压缩的任务书.docx
基于嵌入式量化器和自适应Context建模的ECG信号压缩的任务书一、任务描述心电图(Electrocardiogram,简称ECG)是一种记录心脏电活动的传统方法,通过检测心脏电信号变化来诊断心电图,可以帮助医生及时发现和判断心脏疾病。随着科技的不断进步,ECG信号已被广泛应用于心脏病学、医疗保健等领域。然而,由于ECG信号的特殊性质,其体积非常大,常常需要大量存储和传输的空间,这对于移动设备、远程监测等应用带来了很大的压力。因此,研究ECG信号压缩算法是十分必要和有意义的。本项目旨在实现一种基于嵌入式
基于Context模型的二维ECG信号压缩的中期报告.docx
基于Context模型的二维ECG信号压缩的中期报告一、研究进展情况在基于Context模型的二维ECG信号压缩的研究中,我们主要进行了以下工作:1.数据准备我们使用了MIT-BIHArrhythmia数据库中的100个记录作为研究对象,每个记录包含30分钟的心电信号。这些信号的采样频率为360Hz,每个信号的长度为108000个点。2.信号分割为了使得信号的压缩更加精确,我们对每个记录进行了信号分割,将每个记录分成了多个长度为3秒的片段,即每个片段包含1080个点。每个片段都会被分别压缩。3.Conte