
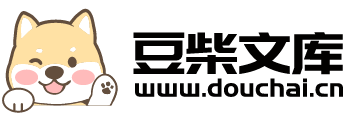
基于混合模型的大规模演化策略的开题报告.docx
快乐****蜜蜂
在线预览结束,喜欢就下载吧,查找使用更方便
相关资料
基于混合模型的大规模演化策略的开题报告.docx
基于混合模型的大规模演化策略的开题报告摘要:混合模型是一种将多种机器学习模型组合起来进行学习的方法,它在实际应用中表现出了强大的性能。而在进化策略中,基于混合模型的策略也表现出了很高的效率和可扩展性。本文针对大规模的演化策略问题,基于混合模型的思路,提出了一种新的演化策略算法。该算法利用了多种不同类型的策略来探索解空间,通过混合模型来学习不同类型策略之间的权重关系和优秀策略的共性,从而有效地提升了演化策略的收敛速度和搜索效果。本文首先介绍了混合模型的基本原理和应用场景,然后详细地介绍了基于混合模型的演化策
基于混合模型的大规模演化策略的任务书.docx
基于混合模型的大规模演化策略的任务书一、背景演化算法作为一种基于生物演化原理的计算模型,在优化问题上具有广泛的应用。其中,演化策略是演化算法中的一种常见形式,它将求解问题视为一个搜索过程,并且使用随机性确定搜索方向。然而,传统的演化策略存在着一些问题,例如容易陷入局部最优、搜索速度较慢等。因此,研究如何改进演化策略的性能具有重要的理论和实际意义。混合模型是将不同的模型组合在一起,以获得更好的性能。在演化策略中应用混合模型可以避免局部最优问题,并提高搜索速度和收敛速度。因此,设计一种基于混合模型的大规模演化
基于排序模型的混合整数演化策略.docx
基于排序模型的混合整数演化策略基于排序模型的混合整数演化策略摘要:混合整数演化策略(MI-ES)是一种用于寻找最优解的进化计算方法。在解决组合优化问题时,MI-ES需要利用排序模型来帮助指导优化过程。本文将介绍MI-ES算法的基本原理及其在排序模型中的应用。通过实验验证,MI-ES在多个组合优化问题中取得了较好的结果,证明了其有效性和可行性。1.引言混合整数演化策略(MI-ES)是一种基于进化计算的全局优化算法,广泛应用于解决组合优化问题。与传统的启发式算法相比,MI-ES具有更好的全局搜索能力和更高的收
基于Penna模型的生物演化模型的开题报告.docx
基于Penna模型的生物演化模型的开题报告1.0研究背景与意义生物演化是生命科学领域中一个重要的研究方向。有关生物演化的许多理论假设、模型和方法已经被提出并推广到广泛的应用领域。其中,Pennacchio(Penna)模型是一种基于个体遗传特征和环境压力的生物演化模型,可以用于模拟和分析生物种群的进化进程。Penna模型的基本思想是将个体的遗传特征表示为二进制位串,并使用该串来对其进行计算和分配能量,从而对其进行仿真。然而,Penna模型还存在许多需要研究和改进的问题。例如,在该模型中,个体遗传特征的选择
基于多目标协同演化算法的大规模自动驾驶策略涌现的开题报告.docx
基于多目标协同演化算法的大规模自动驾驶策略涌现的开题报告一、研究背景随着自动驾驶技术的逐渐成熟和发展,自动驾驶汽车系统已经从单车辆的独立控制转变为多车辆协同控制。在协同控制中,车辆需要考虑到不同的因素,如控制策略、环境信息、路况等等,以保证安全、高效的驾驶。因此,需要寻求一种能够实现多目标优化的演化算法来提高自动驾驶的效率和安全性。二、研究内容本研究将提出一种基于多目标协同演化算法的自动驾驶策略涌现方案。该方案涉及到以下两个方面:1.多目标优化模型的建立针对自动驾驶车辆的复杂环境和不同初始状态,本研究将建