
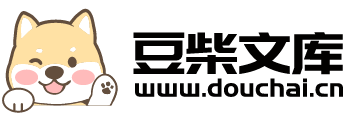
基于机器视觉的田间杂草识别方法研究的中期报告.docx
快乐****蜜蜂
在线预览结束,喜欢就下载吧,查找使用更方便
相关资料
基于机器视觉的田间杂草识别方法研究的中期报告.docx
基于机器视觉的田间杂草识别方法研究的中期报告摘要:杂草是影响农作物生长和产量的主要因素之一,因此,对杂草的识别和控制非常重要。传统的杂草识别方法依赖于人眼观察,不仅效率低下,而且准确性往往受到主观因素的影响。随着机器视觉技术的发展,借助计算机对农田中的杂草进行识别已逐渐引起人们的注意。本报告提出了一种基于机器视觉的田间杂草识别方法,采用深度卷积神经网络(CNN)作为主要的识别模型,并结合区域建议网络(RPN)进行目标定位,实现了杂草的自动识别。实验结果表明,该方法在公开数据集上表现良好,平均准确率高达95
基于机器视觉的杂草识别技术研究的中期报告.docx
基于机器视觉的杂草识别技术研究的中期报告一、研究背景随着农业生产技术的不断发展,机器视觉技术已经成为现代农业生产的一项重要技术。在现代农业生产中,杂草是农民最头疼的问题之一,因此杂草的识别和控制技术也成为了当前研究的热点之一。二、研究目的本研究旨在通过机器视觉技术,实现对杂草的识别和分类,从而为农民提供更加高效、精准的杂草控制方法。三、研究方法本研究采用图像处理和机器学习技术,对杂草的图像进行处理和分析,建立杂草的分类模型。具体方法包括:1.图像数据采集:通过无人机或其他机器设备采集农田中的杂草图像数据。
基于机器视觉的杂草识别图像处理技术研究的中期报告.docx
基于机器视觉的杂草识别图像处理技术研究的中期报告一、研究背景及意义杂草是指影响农作物生长和产量的不受欢迎的植物。它们不仅占用了耕地资源,还会对作物造成竞争性损害,造成粮食作物减产和质量下降。传统的杂草识别方法主要靠人工目测,往往需要大量的人力和时间成本,且精度难以保证。而利用计算机视觉技术进行杂草图像检测,不仅可以提高识别准确率,还可以缩短识别时间,减少人力成本,具有重要的现实意义。因此,基于机器视觉的杂草识别技术研究是当前热门的研究方向之一。二、主要研究内容和方法本研究主要基于机器视觉技术,采用图像处理
基于车载机器视觉的安全带识别方法研究的中期报告.docx
基于车载机器视觉的安全带识别方法研究的中期报告一、研究背景和意义:车载机器视觉系统是近年来汽车安全技术领域的一个重要研究方向。其中车载机器视觉系统识别乘客是否系安全带的技术,能够极大地提高车辆驾驶员和乘客的行车安全,防止事故的发生。二、研究现状:目前,车载机器视觉系统识别是否系安全带的技术已经有了一定的研究成果。主要的研究方法包括基于图像处理和基于深度学习。基于图像处理的方法主要是通过对乘客的头部和身体的图像进行处理,判断是否系安全带。主要的图像处理方法有形态学变换、边缘检测、轮廓提取等。但是,这种方法主
基于卷积神经网络的田间杂草识别方法研究.docx
基于卷积神经网络的田间杂草识别方法研究基于卷积神经网络的田间杂草识别方法研究摘要:杂草对农作物的生长和发展带来诸多危害,因此精确识别和有效管理田间杂草是农业生产的重要问题。基于卷积神经网络(CNN)的田间杂草识别方法,能够通过对田间杂草的图像进行训练和分类,实现自动化的杂草识别。本文首先介绍了卷积神经网络的基本原理和应用,然后详细阐述了基于卷积神经网络的田间杂草识别方法,包括数据集的构建、网络模型的设计、训练和测试等内容。最后,通过实验验证了该方法的准确性和有效性。研究结果表明,基于卷积神经网络的田间杂草