
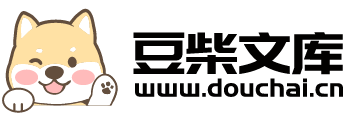
基于支持向量机性能预测的量子遗传网络任务调度研究的综述报告.docx
快乐****蜜蜂
在线预览结束,喜欢就下载吧,查找使用更方便
相关资料
基于支持向量机性能预测的量子遗传网络任务调度研究的综述报告.docx
基于支持向量机性能预测的量子遗传网络任务调度研究的综述报告Quantumgeneticneuralnetwork(QGNN)isanintelligentmethodusedforsolvingcomplexoptimizationproblemssuchastaskscheduling.Thisapproachincorporatestheprinciplesofquantumgeneticalgorithmsandneuralnetworktechniques.ThegoalofQGNNistoach
基于支持向量机性能预测的量子遗传网络任务调度研究.docx
基于支持向量机性能预测的量子遗传网络任务调度研究摘要任务调度是云计算中的重要问题,如何实现有效的任务调度一直是学术界和工业界关注的热点问题。在云计算中,任务调度需要满足多个约束条件,并且需要优化关键指标和性能。本文提出了一种基于支持向量机性能预测的量子遗传网络任务调度方法。通过使用支持向量机训练数据集,对任务执行时间进行预测,并将预测结果用于量子遗传网络中的任务调度。本文通过实验验证了所提出的方法的有效性。实验结果表明,在不同的数据集和工作负载下,所提出的方法具有更好的性能和精度,并且可以满足多个约束条件
基于支持向量机性能预测的量子遗传网络任务调度研究的任务书.docx
基于支持向量机性能预测的量子遗传网络任务调度研究的任务书一、研究背景和意义在云计算时代,任务调度是云计算中一个非常重要的研究和应用领域。在实际的云计算环境中,任务调度需要考虑多种限制因素,如任务执行时间限制、资源约束、网络带宽等因素。随着云计算技术的快速发展,如何高效地调度任务成为了云计算领域的一个重要研究方向。传统的任务调度方法主要基于静态的资源分配方式,这种方法的缺点是缺乏灵活性和适应性。最近的研究,如量子遗传网络、支持向量机等,提供了一些解决任务调度问题的有效方法。支持向量机(SupportVect
基于支持向量机性能预测的量子遗传网格任务调度研究的开题报告.docx
基于支持向量机性能预测的量子遗传网格任务调度研究的开题报告一、研究背景和意义量子遗传网格(QGA)是一类基于遗传算法和网格计算的优化算法,主要用于解决大规模计算问题。在QGA中,任务调度是一个关键的问题,即将多个任务分配到不同的计算节点上,以最大化系统资源利用率和任务完成率。传统的任务调度问题已经得到了广泛的研究,但在QGA中的任务调度问题尚未得到充分的研究。此外,随着云计算和大数据的迅速发展,对于高效的任务调度算法的需求也越来越迫切。因此,研究QGA中的任务调度算法具有重要意义。为了提高任务调度算法的性
基于支持向量机的网络流量预测和资源调度综述报告.docx
基于支持向量机的网络流量预测和资源调度综述报告随着互联网技术的快速发展,网络流量的数据量不断增长,因此越来越重要的是对网络流量的预测和资源调度。支持向量机是一种有效的机器学习算法,已经取得了在网络流量预测和资源调度方面的重要进展。本篇报告将综述基于支持向量机的网络流量预测和资源调度的应用现状、方法与技术、他们的优势和不足以及未来的展望。应用现状:网络流量预测和资源调度是目前网络领域中较为热门的话题。在实际应用中,网络流量预测和资源调度包括多个任务,比如数据收集和处理、特征提取、特征选择、数据集合成、模型建