
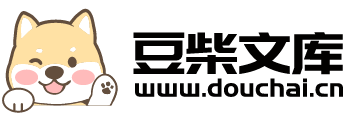
高速RFID射频识别方法研究的中期报告.docx
快乐****蜜蜂
在线预览结束,喜欢就下载吧,查找使用更方便
相关资料
高速RFID射频识别方法研究的中期报告.docx
高速RFID射频识别方法研究的中期报告摘要:本研究致力于探索一种高速RFID射频识别方法。在过去的几年中,RFID技术已经在物流、制造和零售等领域得到广泛应用。然而,目前RFID技术在高速运动物体上的应用还存在一些挑战。因此,我们提出了一种基于相位差分解和深度学习的高速RFID射频识别方法。该方法可以实现高速运动物体的准确识别。在本中期报告中,我们通过对已有的文献进行综述和分析,将目前已有的RFID识别方法分为主动式和被动式两类,并对这两种方法进行了比较和评估。同时,我们也分析了RFID识别中存在的问题和
UHF频段RFID射频前端的研究的中期报告.docx
UHF频段RFID射频前端的研究的中期报告本研究旨在设计和实现一种高性能的UHF频段RFID射频前端,以提高射频信号的灵敏度和稳定性。在中期报告中,我们主要完成了以下工作:1.UHF频段射频前端设计基于现有的射频前端设计方案和技术,我们设计了一种适用于UHF频段的射频前端电路。该电路包括天线匹配电路、低噪声放大器、滤波器以及功率放大器等模块,能够实现对UHF射频信号的接收和传输。2.射频前端性能测试通过对设计的射频前端电路进行性能测试,我们发现该电路的灵敏度和稳定性都比较高。在不同的环境下,射频接收机都能
RFID射频系统防碰撞算法研究的中期报告.docx
RFID射频系统防碰撞算法研究的中期报告一、研究背景随着RFID技术在物流、仓储、供应链管理等领域的广泛应用,RFID射频系统的防碰撞性能成为了研究的热点之一。RFID射频系统的防碰撞指的是在多标签同时进入读写器的范围内,如何避免标签之间的碰撞,保证标签的高效读取和识别。RFID射频系统的防碰撞算法是解决该问题的重要方式之一。二、研究内容本研究的主要任务是对RFID射频系统的防碰撞算法进行研究,通过分析、实验验证和比较相关算法的优缺点,提出一种更加高效的防碰撞算法,以提高RFID系统的可靠性和效率。具体研
UHF频段RFID天线与射频匹配电路研究的中期报告.docx
UHF频段RFID天线与射频匹配电路研究的中期报告中期报告一、研究目的和意义随着智能物联网的快速发展,RFID技术在实际应用中越来越普遍。在RFID系统中,射频信号的传输和接收主要依靠射频天线和射频匹配电路的效率和性能。因此,本研究旨在进一步研究UHF频段RFID天线与射频匹配电路的优化设计,以提高信号传输和接收的效率和性能,推动RFID技术在实际应用中的广泛应用。二、研究内容和进展1.UHF频段RFID天线设计为了优化UHF频段RFID天线的设计,我们进行了多次仿真实验和实际测试。通过分析射频信号的波长
UHF频段RFID射频系统关键技术的研究与设计的中期报告.docx
UHF频段RFID射频系统关键技术的研究与设计的中期报告尊敬的老师:我是您的学生,现就本人的中期报告,向您汇报UHF频段RFID射频系统关键技术的研究与设计的进展情况。一、研究背景近年来,随着物联网技术的发展和应用,RFID系统越来越受到广泛关注。尤其是在物流、供应链、零售等领域,RFID系统已成为必不可少的一种技术手段。而UHF频段RFID系统由于具有较长的读写距离、高的读写速度、强的抗干扰能力等优点,成为了RFID系统中的热门技术。二、研究内容1.UHFRFID标签的研究在UHFRFID系统中,标签的