
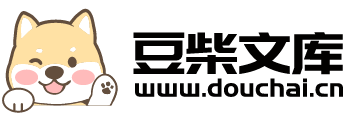
8639790_李朝阳_基于卷积神经网络的脑电信号时频分析理解_基于卷积神经网络的脑电信号时频分析理解V1.docx
代瑶****zy
亲,该文档总共37页,到这已经超出免费预览范围,如果喜欢就直接下载吧~
相关资料
8639790_李朝阳_基于卷积神经网络的脑电信号时频分析理解_基于卷积神经网络的脑电信号时频分析理解V1.docx
PAGE\*MERGEFORMATIIPAGE\*MERGEFORMAT1摘要脑机接口是人工智能的一项重要内容,也是一种全新的大脑与计算机通讯的方式,具有改变人机交互方式的巨大价值。近年来对于脑电信号的研究越来越成熟,许多方法被应用于对运动想象中的脑电信号进行分类。然而卷积神经网络作为一门新兴起的技术,在该领域上应用还比较少。本文探究了卷积神经网络在运动想象脑电信号分类上的应用。主要使用脑机接口竞赛IV2b数据集,探索运动想象脑电信号分类任务。首先查阅了近年来的众多脑电信号分类模型,对于传统机
8639790_李朝阳_基于卷积神经网络的脑电信号时频分析理解_基于卷积神经网络的脑电信号时频分析理解V1.docx
PAGE\*MERGEFORMATIIPAGE\*MERGEFORMAT1摘要脑机接口是人工智能的一项重要内容,也是一种全新的大脑与计算机通讯的方式,具有改变人机交互方式的巨大价值。近年来对于脑电信号的研究越来越成熟,许多方法被应用于对运动想象中的脑电信号进行分类。然而卷积神经网络作为一门新兴起的技术,在该领域上应用还比较少。本文探究了卷积神经网络在运动想象脑电信号分类上的应用。主要使用脑机接口竞赛IV2b数据集,探索运动想象脑电信号分类任务。首先查阅了近年来的众多脑电信号分类模型,对于传统机
8639790_李朝阳_基于卷积神经网络的脑电信号时频分析理解_基于卷积神经网络的脑电信号时频分析理解V1.docx
PAGE\*MERGEFORMATIIPAGE\*MERGEFORMAT1摘要脑机接口是人工智能的一项重要内容,也是一种全新的大脑与计算机通讯的方式,具有改变人机交互方式的巨大价值。近年来对于脑电信号的研究越来越成熟,许多方法被应用于对运动想象中的脑电信号进行分类。然而卷积神经网络作为一门新兴起的技术,在该领域上应用还比较少。本文探究了卷积神经网络在运动想象脑电信号分类上的应用。主要使用脑机接口竞赛IV2b数据集,探索运动想象脑电信号分类任务。首先查阅了近年来的众多脑电信号分类模型,对于传统机
8639790_李朝阳_基于卷积神经网络的脑电信号时频分析理解_基于卷积神经网络的脑电信号时频分析理解V1.docx
PAGE\*MERGEFORMATIIPAGE\*MERGEFORMAT1摘要脑机接口是人工智能的一项重要内容,也是一种全新的大脑与计算机通讯的方式,具有改变人机交互方式的巨大价值。近年来对于脑电信号的研究越来越成熟,许多方法被应用于对运动想象中的脑电信号进行分类。然而卷积神经网络作为一门新兴起的技术,在该领域上应用还比较少。本文探究了卷积神经网络在运动想象脑电信号分类上的应用。主要使用脑机接口竞赛IV2b数据集,探索运动想象脑电信号分类任务。首先查阅了近年来的众多脑电信号分类模型,对于传统机
8639790_李朝阳_基于卷积神经网络的脑电信号时频分析理解_基于卷积神经网络的脑电信号时频分析理解V1.docx
II1摘要脑机接口是人工智能的一项重要内容也是一种全新的大脑与计算机通讯的方式具有改变人机交互方式的巨大价值。近年来对于脑电信号的研究越来越成熟许多方法被应用于对运动想象中的脑电信号进行分类。然而卷积神经网络作为一门新兴起的技术在该领域上应用还比较少。本文探究了卷积神经网络在运动想象脑电信号分类上的应用。主要使用脑机接口竞赛IV2b数据集探索运动想象脑电信号分类任务。首先查阅了近年来的众多脑电信号分类模型对于传统机器学习的方法和深度学习的方法进行了分析然后在实用卷积神经网络的基础上加入深度可分离