
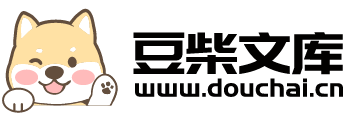
基于SVM的煤与瓦斯突出区域预测研究.pdf
qw****27
在线预览结束,喜欢就下载吧,查找使用更方便
相关资料
基于SVM的煤与瓦斯突出区域预测研究.pdf
第24卷第2期岩石力学与工程学报Vol.24No.22005年1月ChineseJournalofRockMechanicsandEngineeringJan.,2005基于SVM的煤与瓦斯突出区域预测研究南存全1,2,冯夏庭1(1.东北大学资源与土木工程学院,辽宁沈阳110004;2.辽宁工程技术大学资源与环境工程学院,辽宁阜新123000)摘要:支持向量机是20世纪90年代中期兴起的基于结构风险最小化原理的机器学习技术,各项技术性能尤其是泛化能力具有明显优势。基于支持向量机构建了煤与瓦斯突出预测模型。
基于SVM的煤与瓦斯突出预测模型研究的综述报告.docx
基于SVM的煤与瓦斯突出预测模型研究的综述报告煤与瓦斯突出是煤矿安全生产中的主要灾害之一。为了预测和防范煤与瓦斯突出事故,煤矿企业使用了各种方法。其中一种最常见的方法是使用机器学习技术构建预测模型。本文将聚焦于基于支持向量机(SVM)的煤与瓦斯突出预测模型,并对相关研究进行综述。首先,我们需要了解SVM技术。SVM是一种监督学习算法,可用于分类和回归分析。基本思想是将数据映射为高维空间,以找到最大间隔超平面,从而在两个类之间实现最佳分类。SVM在处理高维度和复杂数据上表现优异,并已在许多领域得到应用。针对
基于SVM的煤与瓦斯突出预测模型及应用.docx
基于SVM的煤与瓦斯突出预测模型及应用基于SVM的煤与瓦斯突出预测模型及应用摘要:随着煤矿行业的发展,煤与瓦斯突出事故的发生频率也逐渐增加,给矿工的生命安全和矿山的稳定运营带来威胁。因此,煤与瓦斯突出的预测和预警成为煤矿安全管理的重要任务。本文提出了一种基于支持向量机(SVM)的煤与瓦斯突出预测模型,通过对煤与瓦斯突出事故的数据进行分析和建模,能够准确预测煤与瓦斯突出的风险,为矿山安全管理提供可靠的决策支持。1.引言煤与瓦斯突出是煤矿生产中常见的一种危险情况,其发生往往会导致矿井坍塌,煤炭数据增多,甚至造
基于SVM的瓦斯突出预测研究.docx
基于SVM的瓦斯突出预测研究基于SVM的瓦斯突出预测研究摘要:瓦斯突出是煤矿安全中的一种严重事故,对矿工人身安全构成巨大威胁。因此,研究瓦斯突出的预测方法具有重要意义。本文基于支持向量机(SVM)算法,对瓦斯突出进行预测研究。首先,介绍了瓦斯突出的背景和意义。然后,详细阐述了SVM算法的原理和特点。接着,提出了基于SVM的瓦斯突出预测模型,并利用实际数据进行实验。最后,对实验结果进行分析和讨论,并指出了今后的研究方向。关键词:瓦斯突出,支持向量机,预测模型,数据分析1.研究背景和意义瓦斯突出是煤矿安全中的
基于改进PSO优化LS-SVM参数的煤与瓦斯突出预测研究.docx
基于改进PSO优化LS-SVM参数的煤与瓦斯突出预测研究随着煤矿安全意识的提高和煤矿生产水平的不断提高,预测煤与瓦斯突出已成为煤矿安全管理的重要内容。传统的预测方法存在着一定的缺陷,比如参数选择不合理、准确度不高等问题。针对这些问题,本文提出了基于改进PSO优化LS-SVM参数的煤与瓦斯突出预测方法。首先,介绍了煤与瓦斯突出的概念及其危害性。然后,分别介绍了传统的煤与瓦斯突出预测方法和改进PSO优化LS-SVM参数的煤与瓦斯突出预测方法。传统的煤与瓦斯突出预测方法主要包括基于经验公式、经验参数和单因素分析