
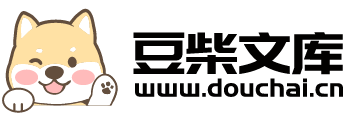
基于Hebb规则的分布神经网络学习算法.pdf
as****16
在线预览结束,喜欢就下载吧,查找使用更方便
相关资料
基于Hebb规则的分布神经网络学习算法.pdf
第30卷第8期计算机学报Vol.30No.82007年8月CHINESEJOURNALOFCOMPUTERSAug.2007基于Hebb规则的分布神经网络学习算法田大新1),2)刘衍珩1),2)李宾3)吴静1),2)1)(吉林大学计算机科学与技术学院长春130012)2)(吉林大学符号计算与知识工程教育部重点实验室长春130012)3)(吉林大学数学学院长春130012)摘要随着知识发现与数据挖掘领域数据量的不断增加,为了处理大规模数据,scalingup学习成为KDD的热点研究领域.文中提出了基于Heb
基于分布式学习的神经网络入侵检测算法研究的中期报告.docx
基于分布式学习的神经网络入侵检测算法研究的中期报告一、研究背景随着互联网的发展和普及,网络入侵事件频频发生,给网络安全带来了极大的威胁。入侵检测技术是网络安全保障的重要手段之一。传统的入侵检测技术往往依赖于专业的安全设备,如防火墙、入侵检测系统等,但这些设备很容易被黑客攻击绕过。为了更加有效地保护网络安全,研究分布式学习的神经网络入侵检测算法显得尤为重要。二、研究目的本研究旨在探究基于分布式学习的神经网络入侵检测算法。具体地,通过构建分布式学习的神经网络模型,将入侵检测任务划分为多个子任务,并采用联邦学习
基于循环学习的模糊神经网络学习算法.pdf
本发明涉及一种基于循环学习的模糊神经网络学习算法,通过定义的“超盒可信度”值来计算每一层超盒扩张后的超盒可信度值;在每一次的全部训练样本的学习过程以后,对所有存在的超盒的分类性能进行分析,删除“可信度低”的超盒,保留“可信度高”的超盒,然后减小最大尺度限制参数值θ,再次进行训练,直到分类器的性能满足要求,或者达到的最小允许取值。本发明既可以避免最大尺度限制参数θ值难以定义的难题,也限制了超盒扩张过程中超盒数目的盲目增加。
基于分布估计算法的BP神经网络优化设计的开题报告.docx
基于分布估计算法的BP神经网络优化设计的开题报告一、选题背景及研究意义BP神经网络被广泛应用于各种模式识别及预测问题中,并在实际工程中取得了较好的效果。然而,神经网络的训练过程常常是一个耗时耗力的过程,并且在一些情况下可能会产生过拟合等问题。因此,如何提高神经网络的训练效率和泛化能力一直是神经网络研究的热点问题之一。分布估计是一种常见的优化算法,它通过对目标函数的梯度估计来实现对函数的优化。与传统的梯度下降算法相比,分布估计具有计算效率高、对初始值不敏感等优点,因此被广泛应用于各种优化问题中。在神经网络的
基于规则模型的分布估计多目标算法及应用的开题报告.docx
基于规则模型的分布估计多目标算法及应用的开题报告开题报告一、选题背景和意义多目标优化是指同时考虑多个目标指标的优化问题,这是现实中大多数决策问题所涉及的问题。在工程优化、金融投资、资源分配等领域中,经常遇到多个目标之间存在冲突或相互制约的问题,如何找到一组最佳的目标取值,使得这些目标同时达到最佳水平成为了一个重要的研究问题。多目标优化算法是解决这种问题的重要工具,常用的多目标优化算法包括遗传算法、模拟退火算法、多目标粒子群优化算法等。在多目标优化中,选择合适的优化算法是非常重要的。传统的优化算法可能无法处