
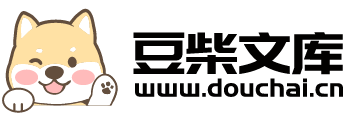
基于bp神经网络的滚动轴承故障诊断方法初探-毕业(论文)设计论文.doc
人生****奋斗
亲,该文档总共23页,到这已经超出免费预览范围,如果喜欢就直接下载吧~
相关资料
基于bp神经网络的滚动轴承故障诊断方法初探-毕业论文设计论文.doc
兰州交通大学毕业设计(论文)兰州交通大学毕业设计(论文)-PAGEII--PAGEI-基于BP神经网络的滚动轴承故障诊断方法初探摘要滚动轴承是机械设备中最常见、应用最广泛的零部件之一,其运行状态对整个设备的工作状态、生产过程都有直接影响。因此对轴承的故障诊断具有非常重要的意义。本文以机械设备滚动轴承故障诊断问题为背景,针对传统的时频分析方法难以全面反映故障信息的缺陷,探讨了BP(BackPropagation,反向传播)神经网络技术在滚动轴承故障诊断中的应用。选取滚动轴承三种故障类型(内圈故障
基于bp神经网络的滚动轴承故障诊断方法初探-毕业论文设计论文.doc
兰州交通大学毕业设计(论文)兰州交通大学毕业设计(论文)-II--I-基于BP神经网络的滚动轴承故障诊断方法初探摘要滚动轴承是机械设备中最常见、应用最广泛的零部件之一,其运行状态对整个设备的工作状态、生产过程都有直接影响。因此对轴承的故障诊断具有非常重要的意义。本文以机械设备滚动轴承故障诊断问题为背景,针对传统的时频分析方法难以全面反映故障信息的缺陷,探讨了BP(BackPropagation,反向传播)神经网络技术在滚动轴承故障诊断中的应用。选取滚动轴承三种故障类型(内圈故障、外圈故障、滚动体故障)下的
基于bp神经网络的滚动轴承故障诊断方法初探-毕业论文设计论文.doc
兰州交通大学毕业设计(论文)兰州交通大学毕业设计(论文)-PAGEII--PAGEI-基于BP神经网络的滚动轴承故障诊断方法初探摘要滚动轴承是机械设备中最常见、应用最广泛的零部件之一,其运行状态对整个设备的工作状态、生产过程都有直接影响。因此对轴承的故障诊断具有非常重要的意义。本文以机械设备滚动轴承故障诊断问题为背景,针对传统的时频分析方法难以全面反映故障信息的缺陷,探讨了BP(BackPropagation,反向传播)神经网络技术在滚动轴承故障诊断中的应用。选取滚动轴承三种故障类型(内圈故障
基于bp神经网络的滚动轴承故障诊断方法初探设计论文.doc
兰州交通大学毕业设计(论文)兰州交通大学毕业设计(论文)-PAGEII--PAGEI-基于BP神经网络的滚动轴承故障诊断方法初探摘要滚动轴承是机械设备中最常见、应用最广泛的零部件之一,其运行状态对整个设备的工作状态、生产过程都有直接影响。因此对轴承的故障诊断具有非常重要的意义。本文以机械设备滚动轴承故障诊断问题为背景,针对传统的时频分析方法难以全面反映故障信息的缺陷,探讨了BP(BackPropagation,反向传播)神经网络技术在滚动轴承故障诊断中的应用。选取滚动轴承三种故障类型(内圈故障
基于bp神经网络的滚动轴承故障诊断方法初探-毕业(论文)设计论文.doc
兰州交通大学毕业设计(论文)兰州交通大学毕业设计(论文)-PAGEII--PAGEI-摘要滚动轴承是机械设备中最常见、应用最广泛的零部件之一,其运行状态对整个设备的工作状态、生产过程都有直接影响。因此对轴承的故障诊断具有非常重要的意义。本文以机械设备滚动轴承故障诊断问题为背景,针对传统的时频分析方法难以全面反映故障信息的缺陷,探讨了BP(BackPropagation,反向传播)神经网络技术在滚动轴承故障诊断中的应用。选取滚动轴承三种故障类型(内圈故障、外圈故障、滚动体故障)下的轴承振动数据,